In the last article, we explained AI for Good and why we need it now more than ever. AI has the potential to accelerate progress across all 17 Sustainable Development Goals (SDGs) by providing scalable solutions for health, education, climate change, and more.
However, a more critical question is: How can we bring more successful AI for Good solutions into the real world?
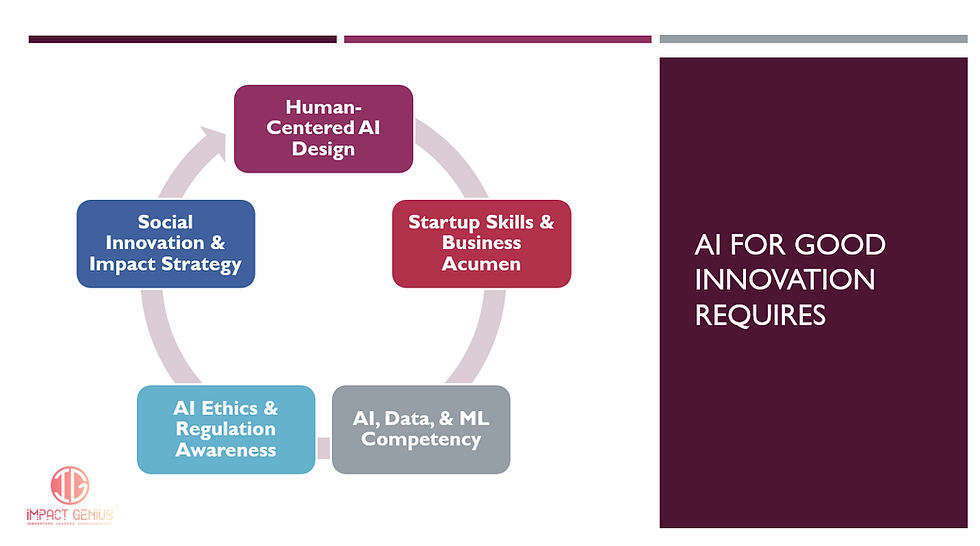
These are the prerequisites for effective AI for Good Innovation.
Without these pillars, an AI for Good solution may not fully achieve the goal of advancing social good.
Human-Centered AI Design
Stanford HAI educators and experts advocate for using a human-centered design approach at every stage of the AI development cycle.
The HAI design approach emphasizes the need to deeply consider the impact on users, community, and society while leveraging interdisciplinary teams and engaging all stakeholders early and often.
Startup Skills & Business Acumen
Did you know that the main reasons 90% of tech startups fail are business-related? While technical challenges also play a factor, key reasons like financing, product-market fit, team skillsets, and operational challenges are at the forefront of why tech startups fail.
Business strategy, therefore, is a core requirement for bringing AI for Good solutions to the real world.
Social Good tech startups, however, navigate added layers of complexity. These include challenges balancing impact with profitability, solving more complex problems, and more. Powerful entrepreneurship skills are required to achieve success across the tech, social good, and business domains.
AI, Data, & ML Competency
Technical expertise is naturally required throughout the AI development lifecycle. However, there are conditions to that technical project leads must remember.
Software engineers and data scientists must collaborate with diverse stakeholders to successfully drive an AI for Good solution. Every step of the AI development cycle in AI for Good requires cross-functional collaboration.
From defining the problem statement to setting the AI project parameters, to data collection and model training, to deploying the solution, evaluating, and iterating:
Every step of the AI development cycle needs various stakeholders at the table.
AI Ethics & Policy Awareness
Ethical AI is foundational in AI for Good.
Practitioners must prioritize privacy, transparency, and explainability at every step of the AI development journey.
Ensuring the solution achieves a positive impact and avoids unintended harm is central to building trust and achieving the project goals.
AI for Good projects often fall in highly regulated industries or across borders. Industry regulation and international implementation require thorough AI policy and compliance planning.
Social Innovation & Impact Strategy
A solid social innovation strategy is required to address the world's greatest challenges effectively. This strategy includes leveraging expert insight to understand the problem space and SDG targets deeply.
It is important to develop an impact strategy that defines how impact will be achieved, measured and demonstrated to stakeholders. Maintaining an agile, iterative approach to course correction rapidly and effectively is also important.
How do we bring more AI for Good innovation to the real world? One of the best ways to do so is to increase the number of AI for Good Startups.
We define an "AI for Good Startup" as a social venture that embeds a commitment to social good within its mission and KPIs, along with business profitability, while leveraging AI in its products or operations.
I believe this is the future of business. Why should you start an AI for Good Startup now? Learn about that in our next blog post.
Comments